Measuring the value of AI/ML-powered assurance
AI/ML powered assurance is fast becoming indispensable for communication service providers (CSPs). As network technologies advance in complexity, so too does the volume of data for assurance processes, encompassing a variety of events, measurements, logs, session records and more. For Network Operation Centers (NOCs) and Service Operation Centers (SOCs) – those tasked with obtaining clear operational insights into networks and services – this presents a host of challenges. Addressing them necessitates automated algorithms that can extract these insights to draw informed conclusions for strategic decision making.
Where does AI/ML-powered assurance deliver value?
Assurance has always been a critical process in CSP operations for the identification, diagnosis and resolution of service-impacting network issues. Now however, by adding AI/ML into the mix, CSPs can also enhance operational efficiencies and significantly boost customer satisfaction and service quality.
Let’s dig deeper into how AI/ML assurance can transform NOC and SOC operations, and how it can contribute to reductions in operational expenditure (opex) and capital expenditure (capex).
Reducing mean time to repair (MTTR)
Traditionally, NOCs and SOCs relied on human operators to monitor systems and manage recovery. But with the emergence of AI/ML technologies, the ability now exists to leverage operational insights to significantly reduce Mean Time To Repair (MTTR). These technologies have introduced a new level of efficiency, with algorithms playing a pivotal role in root cause analysis and automated anomaly detection. Additionally, by leveraging a smarter human-machine interface, powered by the latest GenAI, LLM-based algorithms, efficiency is boosted even further.
Automated healing is yet another tactic to reduce MTTR, enabling human operators to supervise rather than directly manage the healing process. This approach harnesses the power of precise problem analysis, combined with automation by implementing a closed-loop system. And yet, even as this use case rapidly evolves, it’s crucial to acknowledge that not all failures can be accurately identified with current assurance deployments, which might lack access to additional data sources such as social networks, weather reports and traffic information.
Preventing outages
Outage prevention is an additional key factor for improving customer satisfaction. Despite the latest AI/ML prediction algorithms being able to anticipate a considerable number of failure types, the real challenge lies in creating an automated response that can be integrated into the operational process, and provide preemptive solutions to potential failures. It’s effectiveness can be quantified in the overall reduction in outage durations and the reduced number of incidents requiring NOC and SOC intervention.
Supporting service impact analysis
Service impact analysis is a key operational flow that can directly impact service downtime. Considering not every network problem will lead to an outage, it’s crucial to pinpoint and prioritize issues affecting customer services. As a result, by focusing on the affected services and the underlying network CSPs can not only reduce downtime, but also improve both MTTR and customer satisfaction.
Supporting service level agreement (SLA) analysis
In the realm of service level agreements (SLAs), AI/ML-powered assurance is vital in ensuring service quality and reliability. In recent years, the focus on customer SLAs has surged, especially in the enterprise market. CSPs leverage SLAs to differentiate themselves from competitors, notably when serving enterprises and large businesses. This trend is gaining traction with fixed connectivity and layer 2 or layer 3 services, and is also emerging in the mobile domain with the wider rollout of 5G and its SLA-driven capabilities delivered through slice-based services.
Measuring the benefits of AI/ML-powered assurance
AI/ML-powered assurance brings tangible benefits across operational and business spheres. This includes boosting competitiveness by enhancing customer satisfaction and optimizing the use of human and network resources, leading to both reduced opex and capex.
And while the direct impact of assurance might be hard to quantify in areas like customer experience, there are established metrics and key performance indicators (KPIs) for measuring assurance benefits:
- Reduction in alarms
- Fewer trouble tickets
- Shortened mean time to repair (MTTR)
- Less service downtime
- Lower operational effort and cost
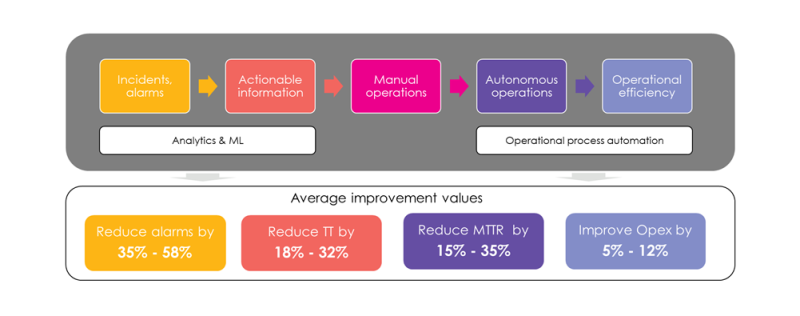
Real-world project results
In a recent project, Amdocs sought to reduce a CSP’s NOC load using Amdocs AI/ML powered assurance. This included deployment of an AI/ML-based root cause analysis algorithm, which successfully learned the patterns of alarm behaviors, clustered symptoms and proposed root causes for network issues. As a result, the symptoms, represented by network alarms, were translated into actionable insights, and subsequently used to enhance manual and autonomous operations.
Outcomes included:
- A 35%-58% reduction in alarms received by the NOC
- An 18%-32% drop in trouble tickets initiated by alarms
- A 15%-35% improvement in MTTR
- A 5%-12% reduction in overall opex effort
Amdocs Helix Service Assurance Suite
Amdocs's market-leading capabilities empower CSPs by delivering superior business results through AI/ML automation. With Amdocs Helix Service Assurance Suite –our intelligent, integrated solution for fault, performance, and service quality management – we place specific emphasis on applying AI/ML and analytics to derive actionable insights that lead to improved operational processes.
For more information, please visit Amdocs Helix Service Assurance Suite.
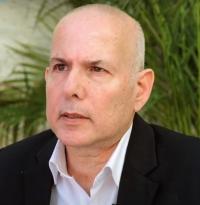
Yuval Stein
Director of Products Technologies & Solutions